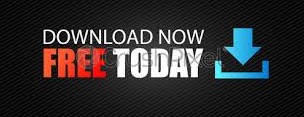
The first method is most convenient and works fine in most cases.
#TEST DATA GENERATOR KERAS ZIP FILE#
In order to do this, you will have to create a shareable link of the zip file in drive. (1) Extract the zip file directly from the drive, as shown below, or (2) use a !wget command to download it to the notebook and subsequently extract it. When you want to import the database into the Colab notebook, there are two ways of doing it. Save the database as a zipped fileĪs per my experience, the best way to store data in Google drive is as a. The generators for train and validation are declared as shown below. The partition for the test set would be similar to the validation set.
#TEST DATA GENERATOR KERAS CODE#
Below is a code snippet to load the train and validation data using the glob module.Īs you can see in the code snippet, the train and validation set is defined inside a dictionary called 'partition'. For examples, '/content/train data/block-id-1/id-label-1.npy'. You can store the labels and training as NumPy files within the same folder. Therefore, below is a function to perform natural sort. For instance, 'id-100.npy' would be placed before 'id-2.npy'. The default sort function in Python is not a natural sort. I generally store 320 examples per block. For example, '/content/train data/block-id-1/id-1.npy'. Hence, it is a good idea to divide your dataset into sub-folders or blocks. If you have thousands of examples, the 'glob' module is not good at loading the files. Store the train, validation, and test data into separate directories. Save individual examples as NumPy arraysįormat each example in the dataset as a separate NumPy array. Below is the definition of a data generator. So, some of my opinions might be biased towards an audio-context. My research is focused on audio classification. Therefore, this post explains some of the dos and don'ts while using data generators with Google Colab. For example, the delay while directly loading files from Google drive. Using data generators with Google Colab was trickier than I expected. Recently, many people have started using Google Colab for machine learning projects. Most of them explain in the context of using a local computer. There are many posts out there that explain the use of data generators. Data generators have two use cases - (1) Data augmentation and (2) loading a dataset that does not fit into the RAM. This way, you can make modifications to the data before feeding it to the neural network or even load it from the secondary memory. Data generators allow you to feed data into Keras in real-time while training the model. This blog post is a tutorial on using data generators with Keras on Google Colab.
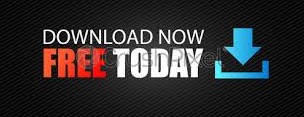